EEG-based action anticipation in human-robot interaction: a comparative pilot study
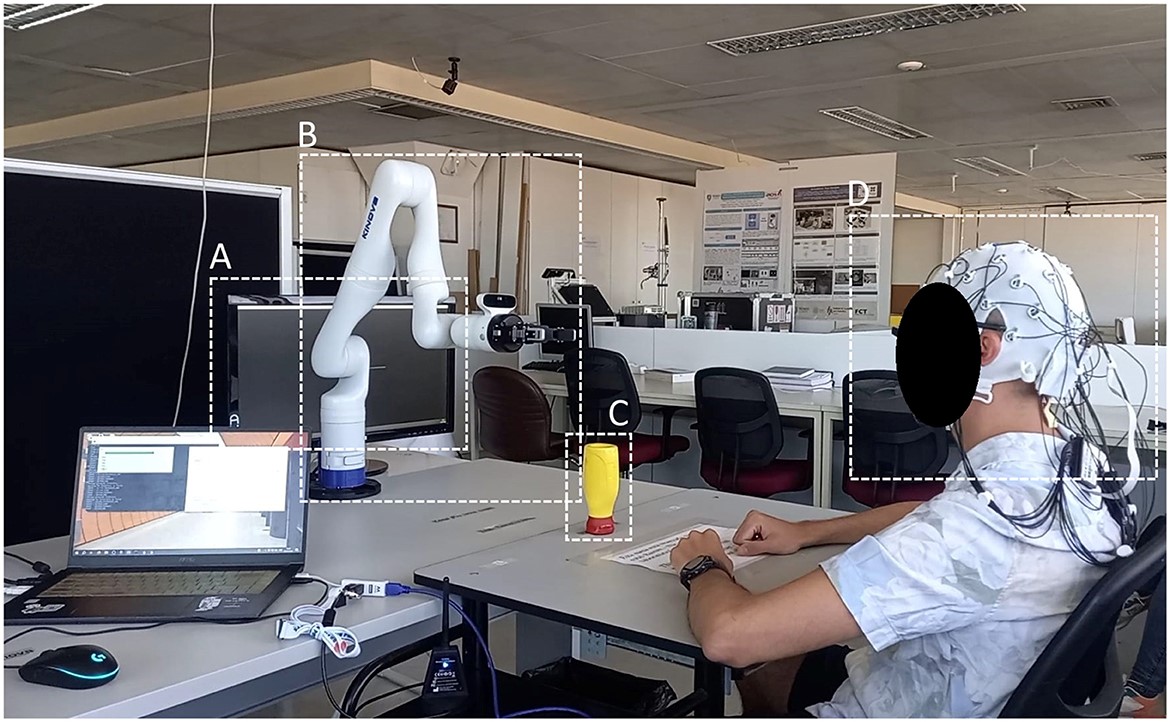
Electroencephalographic (EEG) signals can detect brain activity preceding movement by over a second, enabling predictive capabilities of motor intention. This study explores how EEG can be used for action anticipation in human-robot interaction (HRI), leveraging its high temporal resolution and modern deep learning techniques. We evaluated multiple Deep Learning classification models on a motor imagery (MI) dataset, achieving up to 80.90% accuracy. These results were further validated in a pilot experiment, where actions were accurately predicted several hundred milliseconds before execution. This research demonstrates the potential of combining EEG with deep learning to enhance real-time collaborative tasks, paving the way for safer and more efficient human-robot interactions. See more.