Machine Learning Models to Predicts Biosurfactant Production in Fermentation Processes
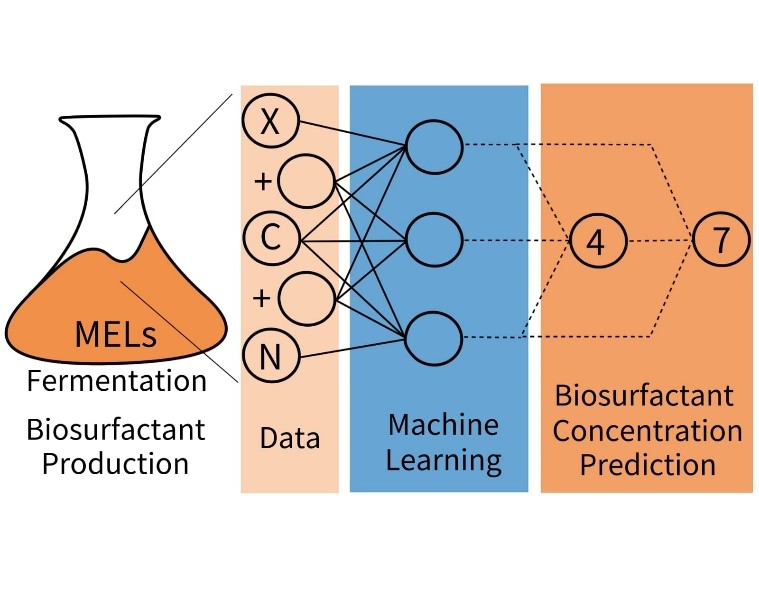
Fermentations are complex and often unpredictable processes, but they generate vast amounts of underutilized data. In a new study published in Applied Sciences, researchers from iBB-Institute for Bioengineering and Biosciences, through a collaboration between the SCERG and 2BRG groups, harnessed Machine Learning to forecast the production of MannosylerythritolLipids (MELs), a high-value biosurfactant, during Moesziomyces spp. cultivation.
Key Highlights:
✔️ Three ML models—Neural Networks (NNs), Support Vector Machines (SVMs), and Random Forests (RFs)—were tested.
✔️ NNs provided the most accurate predictions for days 4 and 7 of the fermentation processs, demonstrating the possibility of obtaining reliable predictions for early batch quality assessment.
✔️ Predictions could reduce costs by enabling timely adjustments or early termination of underperforming batches.
This work paves the way for data driven optimization of biosurfactant production, combining Biotechnology and AI to enhance process predictability. The open-access dataset and code further promote transparency and future advancements.
The study, first authored by Carolina Vares with the support of Sofia Agostinho, was led by Carlos Rodrigues, Nuno Faria with the collaboration of Ana Fred (from IT) and aligns with global efforts to replace petrochemical surfactants with sustainable alternatives. See more.